Concurrent Learning and Resilient Estimation
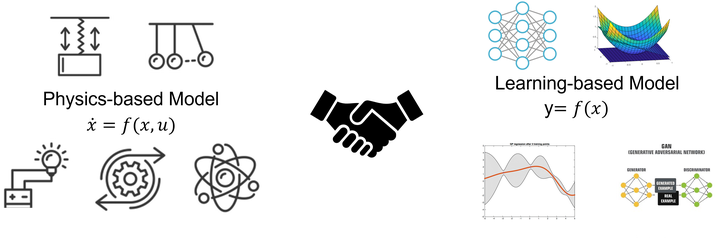
Support prior
It is the estimate of real attack location produced by attack detection and localization algorithm.
The challenge to use support prior in dynamical estimation is:
- the inherent uncertainty of the probabilistic prior information,
- the long training time.
The uncertainty of prior could be modeled by Bernoulli distribution.
Weigthed L1 observer design with prior pruning
A numerical comparison
- Blue line: the fundamental limitation of conventional physics-driven resilient estimators (require more than half of sensors are safe)
- By using the attack detection prior, the estimator could work when 60% sensors are attacked
- With pruning algorithm, that 90% sensors are attacked are acceptable
A simulation
Relevant paper
- Y. Zheng, OM Anubi, Mestha, L., and Achanta, H., “Robust resilient signal reconstruction under adversarial attacks”, American Control Conference. (2023) [Accepted]
- Y. Zheng, OM Anubi, “Attack-Resilient Weighted L1 Observer with Prior Pruning”, American Control Conference. (2021)
- Y. Zheng, OM Anubi. “Attack-resilient observer pruning for path-tracking control of Wheeled Mobile Robot.” Dynamic Systems and Control Conference. Vol. 84287. American Society of Mechanical Engineers. (2020)
- Y. Zheng}, and Olugbenga Moses Anubi “Resilient Observer Design for Cyber-Physical Systems with Data-Driven Measurement Pruning ”, Security and Resilience in Cyber-Physical Systems, Edited by Ali Zemouche and Masoud Abbaszadeh, Springer. (2022)